Haz
09
2020
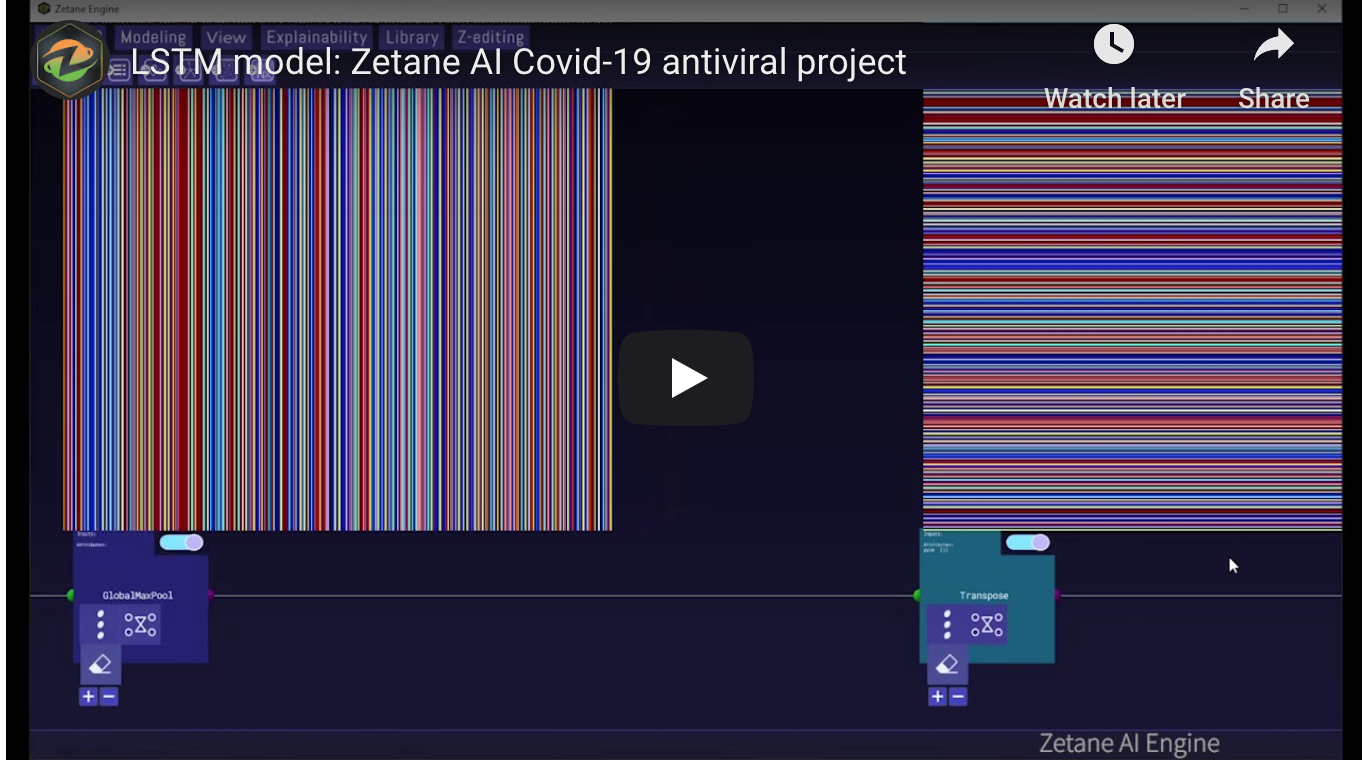
Konuk Yazar
Machine-Learning Driven Drug Repurposing for SARS-CoV-2
We developed artificial intelligence to identify antiviral compounds that merit further study as possible pharmaceutical treatments for COVID-19.
Contributing authors:
Semih Cantürk, Aman Singh, Patrick St-Amant, Jason Behrmann, PhD and fellow colleagues of Zetane Systems
Contact the authors by email at info@zetane.com.
All results here are preliminary and have yet to undergo external peer-review. The findings in this article should not be used to guide clinical decision-making, nor do these findings identify a definitive treatment for COVID-19.
FULL ARTICLE EKTEDİR
FİGÜRLER: https://medium.com/zetane-blog/machine-learning-driven-drug-repurposing-for-sars-cov-2-eedc0a83c38
İndirilebilir Dosyalar